How to Evaluate Your AI Startup Idea: A Founder's Step-by-Step Guide
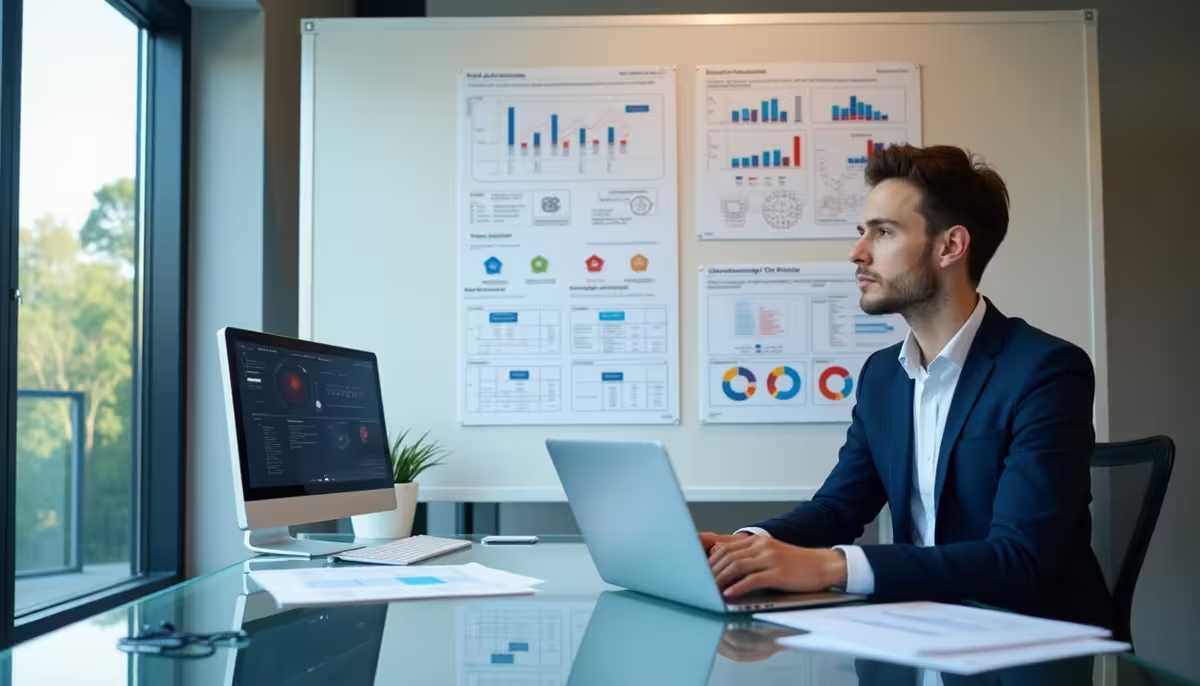
A shocking 42% of startups fail because they create products nobody wants. This statistic represents nearly half of all new ventures that crash before they truly take off.
But artificial intelligence is reshaping the way founders test their startup ideas. AI technology can compress months of market research and analysis into just a few hours. This helps entrepreneurs make informed decisions before they invest their resources.
AI business validation tools have reshaped the startup scene by eliminating guesswork. These tools give founders applicable information about their market potential. We created this complete guide to help you test your AI startup idea properly.
Want to confirm your startup concept with confidence? Let's explore our step-by-step framework that helps you test and refine your AI business idea.
Assessing Technical Feasibility of Your AI Startup Idea
Technical feasibility is the foundation of any AI startup's success. Research shows that 78% of AI/ML projects stop at some stage before deployment. This makes a technical review vital before you invest resources.
Evaluating data requirements and availability
Data forms the foundation of AI advances. Studies reveal that 96% of enterprises face data quality and labeling challenges. About 81% say training AI with data is harder than they first thought. To check if your idea will work, you should:
- Data availability and quality: Do you have clean, relevant data to train AI models? How will you keep a stable data pipeline running for deployment and monitoring?
- Data privacy: AI projects often fail because teams don't think about security needs during data collection
- Data structure: Look at whether your data is structured, semi-structured, or unstructured - this affects model development by a lot
Determining appropriate AI models and algorithms
The right AI models need a balance between performance and practicality. Here's what to look at when picking models:
- Really explore open-source AI models, proprietary models, and third-party solutions
- Filter options based on performances, sizes, and possible risks
- Think about whether a large or smaller AI model fits your specific use case
You should test viable options against your ideal outcome to see which gives results closest to what you need.
Estimating development timeline and technical resources
AI development timelines change based on project complexity. Projects can take anywhere from months to years. Your technical resource plan should look at:
- Infrastructure assessment: Make sure your technology stack—cloud platforms, hardware, and software—can handle AI workloads
- Talent requirements: Look at whether you have (or can get) the right AI talent, toolkits, and development environments
- Budgeting: Start budgeting early to avoid cost overruns, since AI development can cost a lot
Identifying technical differentiators from existing solutions
Now that foundation models are accessible to more people, standing out isn't just about the base technology. You should focus on:
- Vertical knowledge incorporation: Make models better using proprietary data sets for industry-specific use cases
- AI-first product flows: Create fresh product thinking that pre-AI product teams don't deal very well with
- Problem-specific implementation: Go after unsolved pain points that large pre-AI competitors can't address due to contextual limits or organizational inertia
On top of that, review how your technical solution blends with existing infrastructure. Test user acceptance through piloting in the target environment.
Testing Your AI Business Idea Against Market Needs

A brilliant AI startup idea might fail without proper market testing. Your solution needs to address real problems that customers are ready to pay for.
Identifying target customer segments
Finding your ideal customers is the life-blood of market testing. AI has changed how we target audiences. It delivers more accurate client identification and better ROI than old-school methods. AI-driven audience profiling helps us learn about customer priorities, habits, and what drives them.
Your audience segmentation should:
- Use AI to analyze market data and find groups that need your solution
- Build precise audience segments based on behavior and psychology
- Target underserved markets where AI solutions don't work well
The right audience targeting gives businesses a huge edge. It creates offers that resonate with customers and boost conversions.
Validating the problem-solution fit
You achieve problem-solution fit when you find a deep customer problem in an underserved market. This crucial phase requires working with "early evangelists" - customers who will use your solution despite missing features, as long as it fixes their main problem.
Here's how to verify problem-solution fit:
- Run surveys, interviews, and focus groups with potential customers
- Look through online forums and social media for customer feedback
- Let potential customers or industry experts test your AI solution
The real proof comes when early adopters want to pay for your product. If they hesitate, your solution might not address a deep enough problem.
Analyzing competitor AI solutions
AI-powered competitive analysis tools make it easier to understand your rivals, especially when you have limited resources. These tools help spot gaps in existing solutions and show ways to stand out.
AI competitive analysis tools can:
- Monitor competitors' online activities and strategies
- Study backlinks, keywords, and digital footprints
- Use sentiment analysis to understand how customers feel about competitor products
A full picture of your competitors helps you be proactive in the market.
Evaluating Financial Viability and Resource Requirements
Financial planning stands as the vital third pillar when evaluating AI startups, right after technical feasibility and market validation. Your brilliant concept's transformation into a sustainable business depends on understanding money requirements and potential returns.
Calculating development and operational costs
AI development costs change drastically based on complexity and scale. Simple AI models cost around $5,000, while advanced solutions like deep learning models cost nowhere near that - they can cost over $500,000. Training large-scale AI systems with billions of parameters needs investments above $12 million.
Operational expenses typically include:
- Infrastructure costs: Cloud computing and specialized hardware take up 15-25% of your budget. AI startups spend double on compute compared to traditional SaaS businesses.
- Data acquisition: Quality data expenses make up 15-25% of total costs. Complex projects might need over 100,000 data samples.
- Talent: AI engineers and data scientists earn premium salaries that eat up 20-40% of your budget. A small AI development team's cost can reach $400,000 yearly.
Estimating time to market and revenue potential
Time to market affects your competitive edge directly. Every minute counts for AI startups - cutting training time from weeks to days makes a big difference in your bottom line. Quality and speed need careful balance. Launching too early with incomplete models can damage your reputation.
Revenue potential should focus on solid KPIs instead of vague industry claims. These key metrics matter most:
- User growth and retention rates
- Customer acquisition costs
- ROI for early clients
- Monthly Recurring Revenue (MRR) for subscription models
Assessing funding requirements and sources
AI startups secured over $131.5 billion in funding between 2020 and 2024. This shows investors are eager to back AI ventures. Early-stage funding rounds usually range from $10-20 million, sometimes reaching $150 million.
Funding options include:
- Venture capital/private equity: OpenAI raised $6.6 billion in 2024
- Government grants and tax credits: These offer non-dilutive options for innovative projects
- Cloud provider programs: AWS, Azure, and GCP often give discounted services to early-stage startups
- Alternative lending: Revenue-generating startups can get cash-flow based funding
Using AI Tools to Validate Your Startup Concept
AI tools have altered the map of business validation. Unlike traditional approaches, these tools cut down idea validation time from weeks to just hours. This lets founders test their concepts faster before they commit significant resources.
Making use of AI-powered market research tools
AI-powered market research tools combine machine learning, natural language processing, and predictive analytics to gather consumer insights. These tools shine in several key areas:
- Survey automation: Tools simplify research by designing, distributing, and analyzing surveys immediately
- Trend analysis: AI looks at historical and live data to predict consumer behavior with remarkable precision
- Sentiment analysis: These platforms gage public opinion on social media and digital spaces
GWI Spark stands out in this category. It connects users to survey data from almost a million consumers through an easy-to-use chat-based interface. Quantilope smoothly combines AI throughout the research process, from creating surveys to analyzing data.
Testing ideas with AI business validators
AI business validators look at startup concepts and give objective feedback about their potential. ValidatorAI examines your competition immediately and spots unique value propositions competitors might have missed. The system sends you a summary with key points, traction ideas, and resources after your conversation.
IdeaBuddy runs a validation test that measures business idea strength and determines if it's a real chance. DimeADozen analyzes concepts right away, which helps founders concentrate on ideas with market potential and avoid wasted effort.
Getting the most from AI-generated insights
Understanding how these systems analyze information helps you get better results from AI-generated insights. AI models like IBM's granite-34b-code-instruct look at chart data and calculations to spot patterns and trends. They create text-based summaries and highlight key findings.
The best ways to understand AI insights include:
- Your data should be clean and properly contextualized
- Use clear names that match your data accurately
- Check generated insights against original sources
AI-powered insights help founders make informed decisions based on evidence instead of guesswork. This improves their chances of startup success.
Conclusion
Success in AI startups depends on multiple key factors. A proper assessment of technical feasibility, market validation, and financial planning will substantially reduce the risk of creating unwanted products.
Quality data is the life-blood of any AI venture. Teams should secure high-quality datasets, select appropriate models and plan resources before making major investments. Market validation helps confirm real customer needs and financial planning will give a sustainable growth path.
AI-powered tools have made idea validation quicker and more precise than before. These tools can analyze market trends, test business concepts and provide useful insights that turn months of research into hours of analytical decision making.
Your journey toward AI startup success begins with a complete evaluation. The right tools, methodologies and insights covered in this piece will help you assess your startup idea's potential and boost your chances of building a thriving AI venture.