How to Validate Startup Ideas with AI: A Step-by-Step Framework That Works
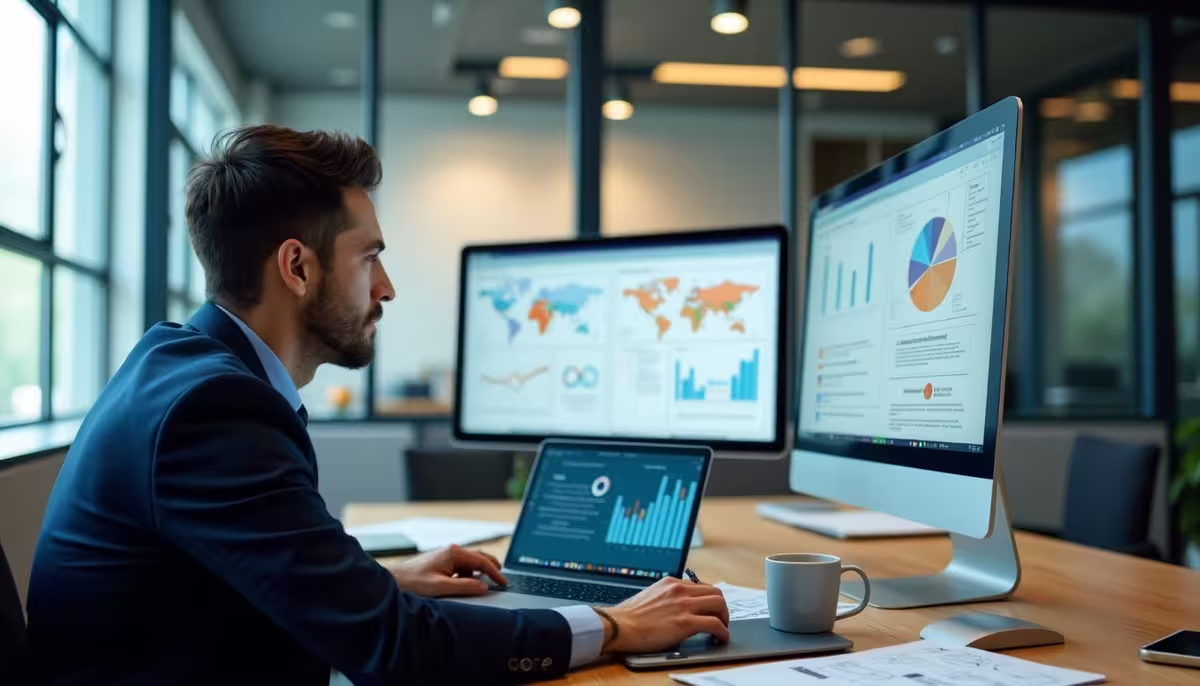
Did you know it takes about 40 hours of research to prove a startup idea right? But now you can complete this significant process in just one hour with an AI business idea validator.
AI startup ideas are changing the way we test business concepts. AI agents and programmable micro-services help us review market demand, technical requirements, and business strategies more efficiently than ever before.
We created this complete framework to help you test your startup idea with AI. This piece will show you how to use tools like ChatGPT and GoZigZag to generate and test business opportunities. It's perfect if you want to test a business idea or need a well-laid-out approach.
Want to see how AI can change your startup validation process? Let's take a closer look at our step-by-step framework that gets results.
Understanding AI Tools for Business Idea Testing
AI business validation tools have changed how entrepreneurs test their ideas. Gone are the days of spending months to gather market feedback or thousands on focus groups. AI now gives evidence-based results within minutes or seconds.
Types of AI validation tools available today
The marketplace now has specialized AI tools that can verify almost every aspect of an idea:
- Market Analysis Tools: ValidatorAI gives detailed feedback on market chances, legal considerations, and customer segments within minutes. DimeADozen creates thorough business reports with market trend analyzes and financial forecasts.
- Rapid Validation Platforms: Founderpal.ai gives quick feedback and verifies business ideas in just 10 seconds—a great way to get help for early-stage startups. GoZigZag improves lean canvases and customer validation questions from a single-sentence business concept in about 90 seconds.
- Specialized Validators: Tools like Checkmyidea analyze customer sentiment, while RebeccAi handles financial projections and business plan creation.
How AI compares to traditional validation methods
Traditional validation usually needs time-heavy market research, expensive focus groups, and biased human analysis. AI validation brings major improvements:
AI can verify a business idea in just 72 hours instead of weeks or months with old methods. The costs are lower too—according to McKinsey, AI can cut validation costs by up to 83%. AI's accuracy stands out as it processes big datasets to spot patterns that humans might miss.
On top of that, it brings real results: 63% of companies saw revenue grow by 5% or more, while 32% reduced costs by 20% or more year-over-year.
Key capabilities of AI in startup validation
AI adds several powerful features to the validation process:
AI algorithms process huge datasets including social media trends, search data, and consumer behavior analytics to spot market needs. AI uses natural language processing to study customer feedback and measure public sentiment toward product ideas.
AI excels at tracking competitors' online presence, market share, and strategies. It can run business scenarios and predict outcomes to help founders understand if their idea will work financially.
AI validation tools turn complex data into useful insights through user-friendly dashboards. Entrepreneurs can quickly grasp market dynamics and make smart decisions.
Preparing Your Startup Idea for AI Validation
My startup concept needs proper structure before I use AI validation tools. A clear idea will lead to more accurate AI validation results. Here's how to prepare effectively.
Defining your core business concept clearly
The first step is to express my business concept with precision. I need to document the problem my startup solves, my solution, and the value customers will receive. Tools like GoZigZag can generate a lean canvas and validation questions in about 90 seconds from a single-sentence business concept. The quality of these outputs depends on how well I define my core concept.
Identifying key assumptions to test
My business idea rests on critical assumptions that need testing. These assumptions fall into five categories:
- Desirability assumptions: Will customers actually want this solution?
- Viability assumptions: Can this idea generate eco-friendly revenue?
- Feasibility assumptions: Can we build and execute this solution?
- Usability assumptions: Will customers know how to use this effectively?
- Ethical assumptions: Could this solution cause potential harm?
The next step is to prioritize these assumptions based on their importance and current evidence. This helps target AI testing toward the most critical unknowns.
Creating effective AI prompts to get better results
The final step focuses on crafting precise AI prompts that give applicable information. AI platforms like ChatGPT provide validation results based on prompt quality. Good prompts should:
- Specify my role request (e.g., "Act as a market research analyst")
- State the business concept clearly
- Ask specific questions about key assumptions
A targeted prompt works better than general questions. You might ask: "Analyze the market demand for [my product] among [specific demographic] solving [specific problem]"
Good preparation will help AI validation tools give meaningful feedback instead of generic responses.
Step-by-Step AI Validation Process
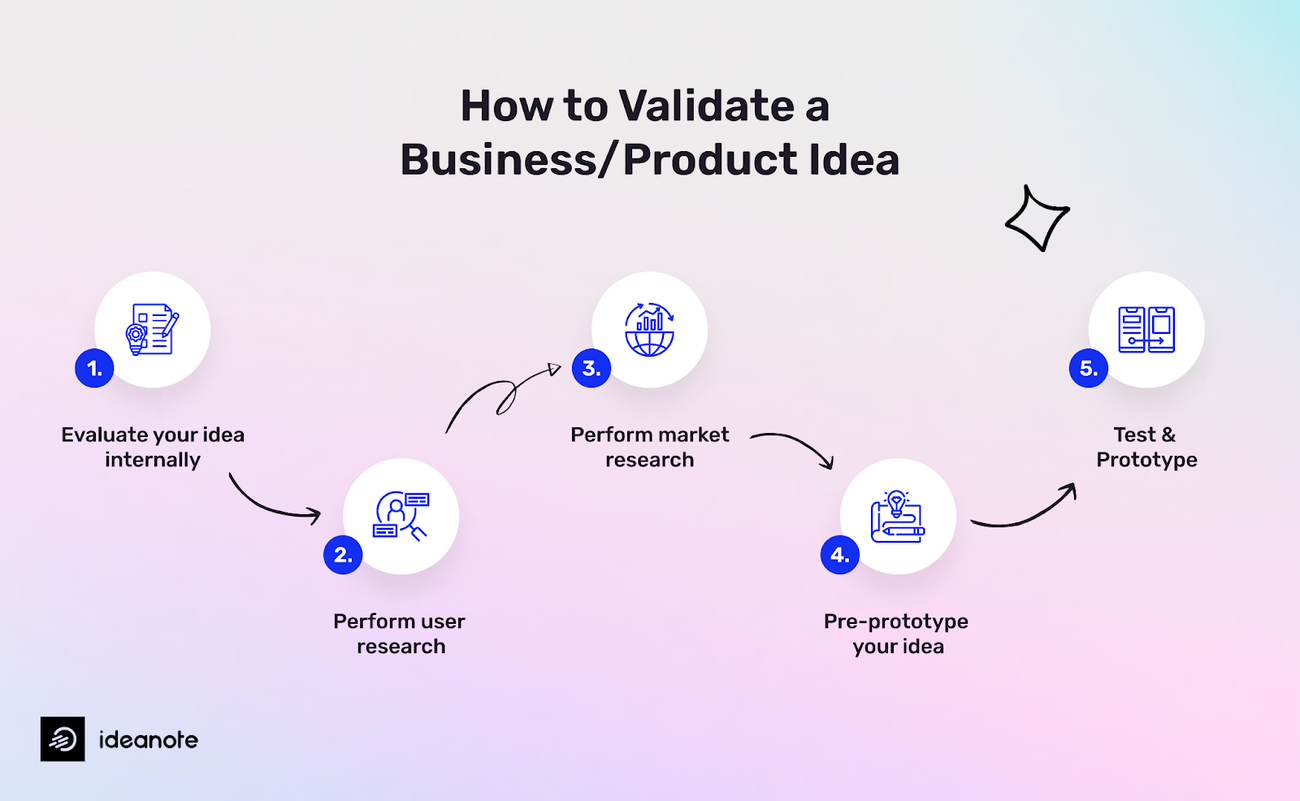
My startup concept is ready. The next step involves proving it right through AI. This approach helps me look at my idea from different angles to check if it works.
Market analysis using AI tools
AI-driven market research tools AI-driven market research tools help analyze immediate data about how customers behave. These tools scan thousands of customer reviews, support tickets, and social media discussions to spot common pain points. To name just one example, FinTrack used ChatGPT to check customer feedback and found major issues before development started. AI also processes location data and shows unexpected patterns about where customers are and which markets remain untapped. Tools like Foresight AI collect data about consumer behavior trends and prove the demand for new product lines.
Technical feasibility assessment
The technical feasibility check looks at computing resources, software tools, and infrastructure needed to build the product. This phase checks if the data is ready and good enough because AI systems need quality data to make accurate predictions. Studies show that while we can automate 80% of vision tasks technically, only 18% make economic sense at current costs. This step balances what's technically possible against real challenges.
Financial projections and viability testing
The money side of validation uses AI to create accurate forecasts by looking at past sales, market trends, and economic indicators. AI forecasting shows what's coming next and helps avoid budget surprises and cash problems. While AI can't predict the future perfectly, it finds patterns in financial data. These patterns create best-case, worst-case, and normal scenarios that help plan hiring, manage cash, and adjust marketing costs. Small businesses and startups can now use AI financial modeling that works like having a team of junior analysts.
Competitive landscape mapping
Competitive analysis shows how rivals think and position themselves. AI tools like Kompyte watch what competitors do across platforms. They show price changes, product updates, and new features. Crayon points out key competitors making waves in the industry. ChatGPT learns about past data and market trends to give detailed insights about risks and opportunities. AI excels at finding patterns in how competitors price things and run promotions. This helps create plans before problems happen instead of reacting to them.
Interpreting and Acting on AI Validation Results
Making sense of validation data from AI tools becomes the deciding factor in your startup's success. Let's get into how you can interpret this information and take the right steps.
Understanding confidence scores and data reliability
AI validation tools typically provide confidence scores—numerical indicators ranging from 0 to 1 that reflect the statistical certainty of predictions. Most business decisions need a confidence score of 80% or higher. Products in sensitive areas like finance or medicine should want scores close to 100%.
Note that confidence scores depend on your data quality. Low confidence scores might point to problems like poor input document quality or inconsistent data types. Your AI models need high-quality data to generate accurate predictions, relevant recommendations, and intelligent automation.
Identifying areas for idea refinement
Feedback is raw data that needs careful analysis. Look for patterns, spot common themes, and focus on key insights. The MoSCoW framework gives you a well-laid-out approach:
- Must-have: Essential fixes or features
- Should-have: Important but not urgent
- Could-have: Optional enhancements
- Won't-have: Ideas that don't arrange with current goals
Small, controlled iterations play a significant role to test improvements. Roll out updates to some users and track the effects through metrics like participation or error reduction.
The right time to pivot or proceed
Hard data often tells you when to change direction. A consistent lack of interest or realizing your idea won't grow as big as you thought might mean it's time to pivot. Most ideation pivots happen within three months of launch, while major pivots usually come around the one-year mark.
Sometimes staying the course makes sense if your product shows promise despite challenges. Dalton Caldwell from Y Combinator explains that pivoting comes down to opportunity cost—getting "more shots on goal" to find product-market fit. Quick decisions help you move faster and keep adjustment costs low.
Conclusion
AI-powered startup validation marks a radical alteration from traditional methods. It saves precious time and resources while providing analytical insights. My research and experience show that successful validation relies on three vital elements: proper preparation, systematic testing, and careful interpretation of results.
A well-defined business concept helps AI tools generate meaningful insights instead of generic feedback. The testing process across market, technical, and financial dimensions helps spot potential challenges before major investment.
AI validation tools let you gather and analyze validation data quickly for faster decision-making. The results might suggest moving forward with the original idea or pivoting to a new direction. These tools give you the confidence to make informed choices.
Keep in mind that AI validation tools work best as part of an all-encompassing approach. Your startup will have the strongest foundation when you combine AI-generated insights with human judgment and industry expertise.