How to Do AI Startup Market Research: A Founder's Step-by-Step Guide
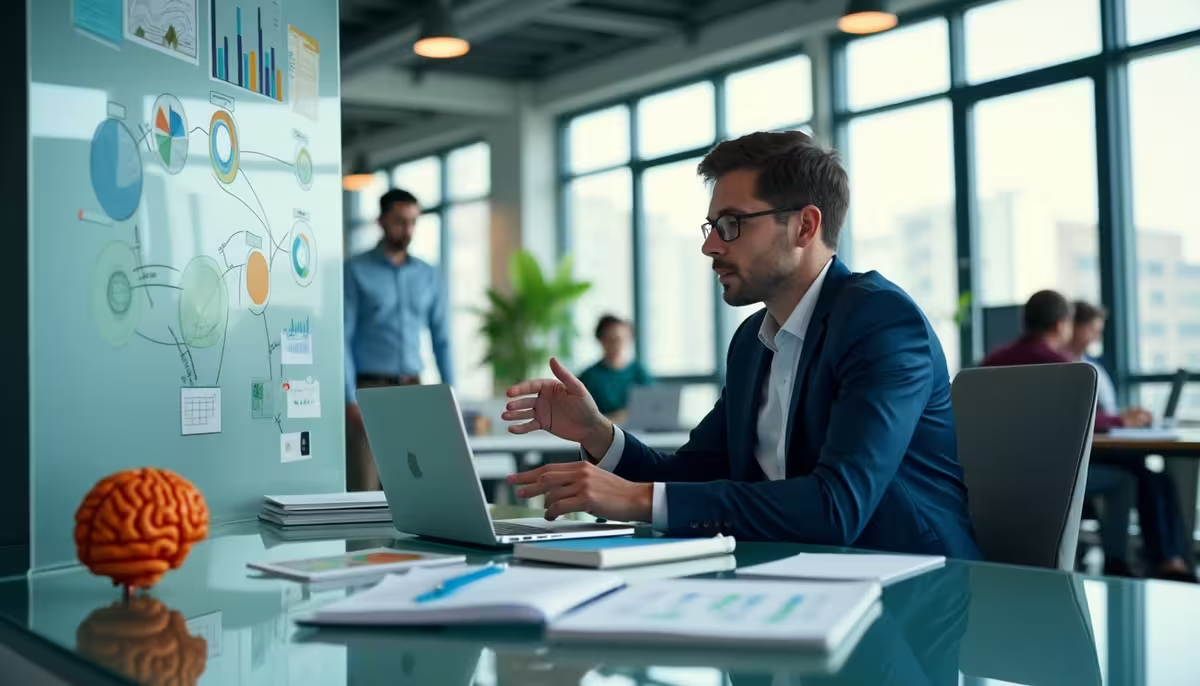
Here's a striking fact: 90% of the world's data has been created in just the last two years, and we generate over 2.5 quintillion bytes each day.
This remarkable surge makes AI startup market research both thrilling and complex. AI market research tools have revolutionized the process. These tools analyze data 100 times faster than traditional methods that could take weeks or months. This gives startup founders like us much deeper understanding of our markets.
The numbers tell an impressive story. The global market research industry stands at $87.7 billion and will grow by another $15 billion within four years. Artificial intelligence powers this expansion with remarkable results. Companies that use AI for predictive analytics have improved their decision-making accuracy by 20% and boosted operational efficiency by 30%.
We created this detailed guide to help you understand the AI startup landscape, verify your market potential, and set your startup on the path to success. Let's take a closer look at the key steps to conduct effective market research for your AI startup.
Understanding the AI Startup Landscape
The AI market has seen incredible growth, with global investments hitting USD 92 billion in corporate funding during 2022. One-third of organizations now use generative AI in at least one business function regularly.
Current state of AI market
Companies are adopting AI at unprecedented rates. About 40% of organizations plan to boost their AI investments because of advances in generative AI. The market shows strong performance in three areas: marketing and sales, product development, and service operations. On top of that, it brings real benefits - companies using AI see 42% lower costs and 59% higher revenue.
Key AI startup segments
AI startups fit into three main categories:
- Core AI Companies: These startups build foundational technology that boosts AI creation and deployment processes
- Application AI Companies: They create tools that help businesses perform specific tasks using AI in different industries
- Industry-Specific AI Companies: They use AI to solve particular business problems within specific verticals
Healthcare, finance, and retail sectors lead the way. The banking sector could add up to 5% value through AI implementation. Industries that rely heavily on knowledge work show higher potential for disruption and value creation.
Market size and growth projections
The global AI market stands at USD 196.63 billion in 2023 and should grow at a strong CAGR of 36.6% through 2030. The generative AI segment could add up to USD 15.70 trillion to the global economy by 2030.
Regional patterns show North America leading with a 30.9% market share, thanks to the United States' strong AI ecosystem. The Asia Pacific region holds 25.6% of global revenue and shows great growth potential. AI startups secured over USD 100 billion in funding between 2020 and 2023, showing strong investor confidence.
The market should reach USD 1,339.10 billion by 2030. Better computational power, more data availability, and rapid digital transformation drive this growth. The healthcare sector stands out and should gain a leading market share by 2030.
Identifying Your Target Market
Your AI startup's success starts with a clear picture of your target market. Good market research helps us learn about and confirm who our ideal customers are.
Define your ideal customer
An Ideal Customer Profile (ICP) helps arrange our sales and marketing efforts with the right audience. Your ICP shows which companies get the most value from our AI solution. You should think over these key aspects while developing an ICP:
- Company size and revenue thresholds
- Industry and business type
- Geographic presence
- Organizational complexity
- Technical maturity level
Research proves that companies with well-laid-out ICPs get better business results by a lot. We must keep updating our ICP to stay in sync with changing market conditions and business direction.
Map out use cases
The best original AI use cases should show high value with low complexity. Here's what to look for while mapping potential applications:
- Focus on operational priorities
- Identify data-rich areas
- Ensure executive sponsorship
- Arrange with business metrics
To name just one example, AI customer segmentation has shown remarkable results - businesses using AI-driven segmentation report 42% cost reductions and 59% revenue increases. Predictive analytics also makes it possible to forecast future customer behaviors and priorities, which leads to better targeting.
Confirm market need
Market validation proves customer interest before we commit resources to development. This process helps us:
- Watch real user behavior instead of relying on hypothetical claims
- Test assumptions through digital and physical experimentation
- Measure actual results using up-to-the-minute data analysis
- Set priorities based on analytical insights
Studies show that companies using AI-powered solutions make 20% more accurate decisions. In spite of that, your solution should address these key questions before development:
- What specific customer problems does it solve?
- Are there like products in the market?
- Will customers pay for the solution?
- Is it financially viable?
Analyzing AI Market Competition
AI startup success depends on understanding the competitive landscape. New data reveals AI startups got a record 46.4% of total venture capital funding in 2024, reaching USD 209 billion.
Research existing AI solutions
The AI market has three main types of competitors:
- Tech Giants: Google, Microsoft, and IBM dominate with detailed AI solutions. IBM's Watson platform earns USD 61.90 billion yearly. Google provides advanced services that include video analysis, speech recognition, and multi-language processing.
- Specialized AI Companies: DataRobot and H2O.ai focus on specific niches. DataRobot guides automated machine learning solutions. H2O.ai stands out in cloud-based predictive analytics.
- Emerging Startups: Scale AI and Databricks bring innovative solutions to complex problems. Scale AI's expertise lies in data labeling for machine learning models, which is definitely essential for AI development.
Study funding patterns
AI startup funding shows remarkable growth. Global venture funding for AI startups hit USD 131.50 billion in 2024, showing a 52% increase from last year. North America leads with almost a third of deals and 60% of VC investment value.
Key funding trends show:
- Regional Distribution: The USA leads global AI funding, with 7 out of 10 top AI investing companies based there.
- Corporate Investment: Intel, Alphabet, and Microsoft actively invest in AI startups.
- Sector Focus: Healthcare AI received USD 5.60 billion in investment. Fintech AI solutions are expected to reach USD 70.10 billion by 2033.
New AI startup founders should know that mega-deals over USD 1 billion shape current funding trends. But all but one of these large deals show AI-focused firms gained a bigger share of VC investment value in 2024 compared to previous years.
Validating Technical Feasibility
Technical validation is the life-blood of any successful AI startup venture. Recent studies show AI makes decisions through algorithms that either follow rules or review large quantities of data to identify patterns.
Assess AI capabilities
A systematic approach helps evaluate AI capabilities and ensures reliable performance. Machine learning consists of multiple layers, which makes it more complex than traditional rule-following computing. The proper assessment requires:
- AI models need thorough testing across performance dimensions
- Training data should line up with operational environments
- Model architecture should capture salient operational features
Research shows 40% of AI adopters report low or medium sophistication across data practices. Startups can overcome this challenge by focusing on quality evaluations that measure their model's prediction accuracy against labeled test data.
Review data requirements
Data quality directly affects AI model performance. Industry analysis shows training data sets are the foundations of any AI model and they end up dictating the accuracy of results. Key factors include:
- Data Volume and Quality: Small data volumes limit prediction accuracy. Projects need sufficient training data to refine outcomes and remove biases.
- Infrastructure Requirements: AI systems need reliable data storage and management solutions that can handle high volumes while maintaining data quality and fast access.
- Regulatory Compliance: GDPR and privacy legislation limit data sharing and automated decision-making capabilities. Companies have responded - 70% confirmed creating new roles specifically to manage GDPR-related compliance.
- Technical Resources: AI model training needs significant computational power. Organizations must ensure their project scope lines up with available resources. Cloud capabilities become necessary as the elasticity and services in cloud environments enable these technologies to exist.
Startups can position themselves better for technical success by carefully thinking about these requirements. Good data validation ensures datasets meet predefined standards and project requirements, which leads to better-performing models.
Conclusion
Market research forms the backbone of every successful AI startup. Our detailed analysis shows that the AI market presents amazing opportunities for businesses of all sizes. The market is expected to reach USD 1,339.10 billion by 2030.
Your success will depend on three key elements. A clear picture of your target market will help you arrange your solution with what customers actually need. A full picture of your competition will reveal untapped opportunities in the market. Technical validation will ensure your AI solution provides real value while meeting data and infrastructure needs.
Effective market research must dig deeper than basic analysis. Your startup will thrive when you monitor markets regularly, validate your assumptions and adapt quickly to customer needs. These insights combined with a well-laid-out approach will position you to build an AI startup that meets market demands and creates lasting value.